Missed a talk? No worries! Watch the recordings anytime at https://bit.ly/LSNI-2024-videos
For safety, please register via our Zoom link (https://bit.ly/LSNI-2024), using your affiliation email when possible.
Once registered, you’ll automatically receive notifications for upcoming talks one week in advance.
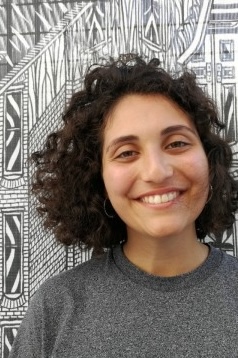
The emergence of social identity
by Melody Sepahpour
02.05.2024 11am CET
Abstract. This study investigates the emergence of a social identity among individuals critical of COVID-19 vaccine policies in France. Amidst concerns over potential restrictions and obligations linked to the vaccine, a collective of individuals has convened both offline and online to vocalize their dissent and affirm their autonomy in vaccination decisions. Perceived as deviant by mainstream media, government, and society at large, this analysis explores the group’s emerging identity in response to unfolding events. Using computational analysis of tweets spanning the year following the vaccination campaign, this research explores how metrics including cosine similarity, pronoun usage, and outgroup labeling elucidate the emergence of social identity. Our findings reveal a pivotal shift in user engagement and identity dynamics following President Macron’s announcement mandating vaccination for health workers. Our results showed significant changes in linguistic patterns, suggesting the crystallization of a social identity in response to rejection. Furthermore, we identify a core group of proficient and consistent users who play a central role in fostering group cohesion. A focused examination surrounding Macron’s speech unveils how new entrants adapt their linguistic expressions to align with the emergent group identity. These findings shed light on the intricate mechanisms driving social identity formation amidst contentious public health policies, offering insights into the dynamics of online discourse and collective action.
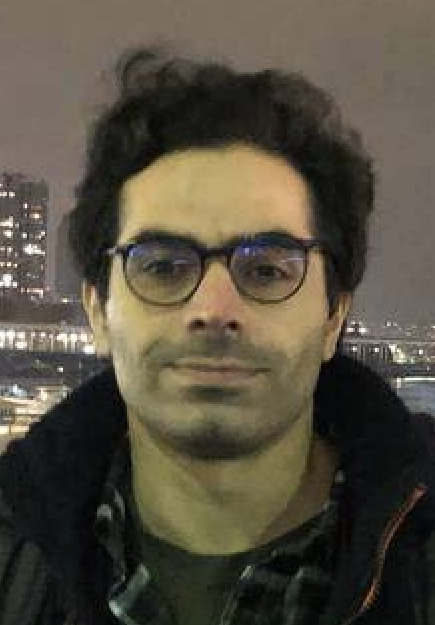
Network heterogeneity explains spatial heterogeneity in the ecology of pathogen strains
by Pourya Toranj Simin
23.05.2024 11am CET
Abstract. The homogeneity assumption in epidemiology assumes that all elements within a system share similar characteristics, which can oversimplify models and predictions. However, real-world heterogeneity significantly influences outcomes, especially in epidemiology, where various sources of heterogeneity impact infectious disease circulation.
In this presentation, I will discuss the impact of heterogeneity in social contact patterns on pandemic dynamics, focusing on COVID-19 variants. Early in the pandemic, variants of concern (VOCs) emerged, with increased transmissibility and immune escape, but competition dynamics varied across locations. We investigated how social contact networks and degree distribution heterogeneity affect variant co-circulation. Computational modeling revealed that the advantage of interacting variants is sensitive to population properties, with high dispersion in degree distribution slowing down the emergence of more transmissible variants, while accelerating the diffusion of variants escaping immunity. I will also discuss how we could validate our findings through empirical analysis using proxy data on contact networks.
Past Talks
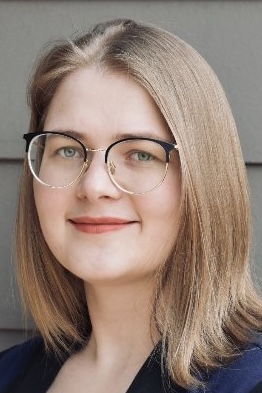
Modeling misperception of public support for climate policy
by Ekaterina Landgren
25.04.2024 4pm CET
Abstract. Mitigating the consequences of climate change and reducing political polarization are two of the biggest problems facing society today. These problems are intertwined, since meeting international climate-mitigation targets requires implementing policies that accelerate the rate of decarbonization, and these policies can succeed only with widespread bipartisan support. Since the late 1980s, climate change has become a strongly polarizing issue in the United States. However, overall support for climate policy is high, with 66-80% of Americans supporting climate policies. Curiously, 80-90% of Americans underestimate public support for these policies, estimating the prevalence of support to be as low as 37-43%. (Sparkman et al. Nature communications 13.1 (2022): 4779.) The implications of such widespread misperception range from individual behaviors to legislative outcomes. Supporters of climate policy are more likely to self-silence if they believe their peers do not support it, and politicians are less likely to promote policies they believe to be unpopular. Here we present an agent-based social-network model of public perception of support for climate policy grounded in previous empirical studies and opinion surveys. We find that homophily effects alone do not explain widespread misperception. However, our network analysis suggests that disproportionate representation of opposition to climate policy among central nodes can offer a potential explanation for underestimation of public support. In order to assess the validity of this assumption in the real world, we explore the coverage of climate policy in U.S. news media in order to inform our model.
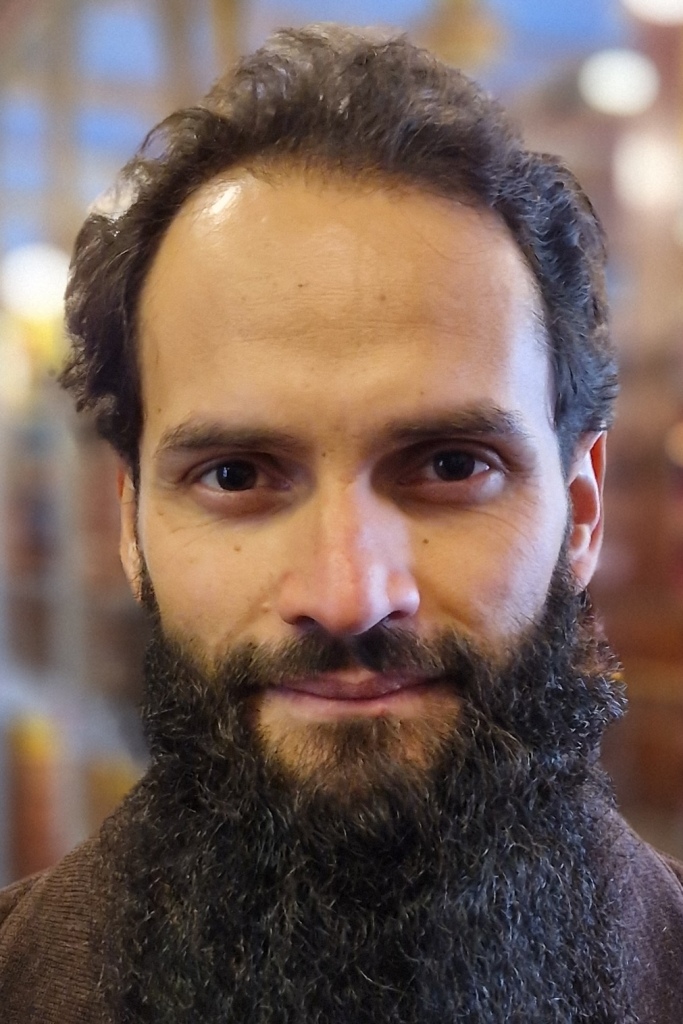
Quantifying spatial heterogeneity and urban segregation on networks through diffusion
by Sandro Sousa
11.04.2024 11am CET
Abstract. Socioeconomic segregation has an important role in the emergence of large-scale inequalities in urban areas. Most of the available measures of spatial segregation depend on the scale and size of the system under study, or neglect large-scale spatial correlations, or rely on ad-hoc parameters, making it hard to compare different systems on equal grounds. In this talk, I will show a family of non-parametric measures for spatial distributions, based on the statistics of the trajectories of random walks on graphs associated with a spatial system. These difusion-based quantities provide a consistent estimation of segregation in synthetic spatial patterns, and they were used to analyse the ethnic segregation of metropolitan areas in the US and the UK. They also used to understand the disproportionate incident of COVID-19 cases among African Americans. This approach, as measured through diffusion on graphs, allows us to quantify and compare sociospatial phenomena in urban areas having different sizes, shapes, or peculiar microscopic characteristics.
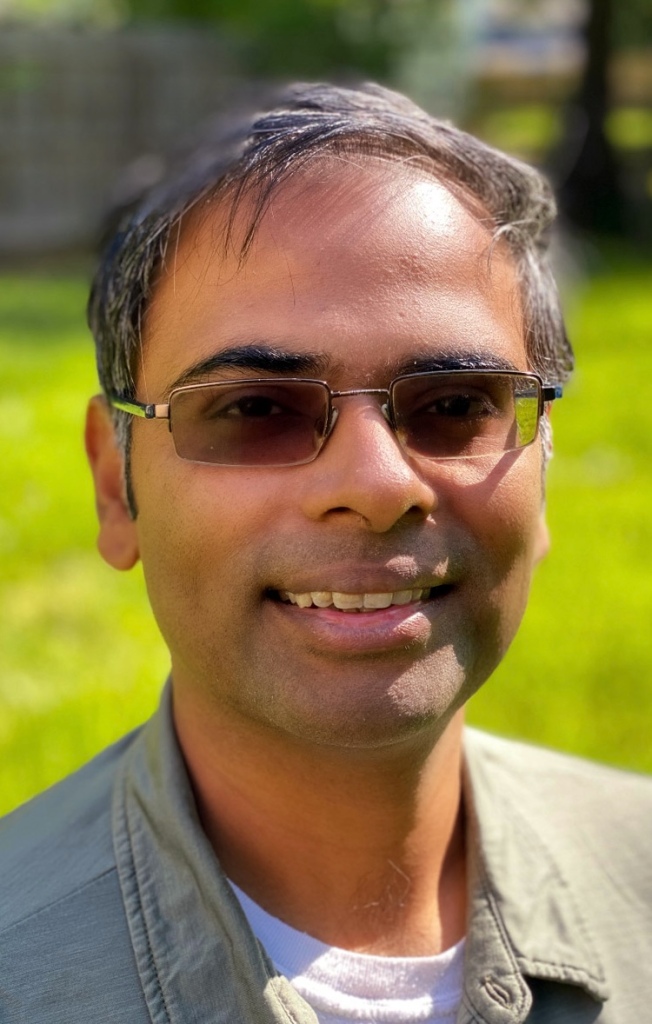
Surprising phenomenon in the math of network models
by Shankar Bhamidi
28.03.2024 2pm CET
Abstract. The goal of this talk is to both convey the importance of domain experts (like those involved in the current initiative) to guide mathematicians like myself in their choice of problems, as well as convey the importance of math techniques in understanding proposed network models, through three personal research experiences: 1. In the context of one fundamental static model in social networks, the exponential random graph model (ERGMs) we will describe what math probability says in one specific (“ferromagnetic”) regime (joint work with Allan Sly and Guy Bresler). 2. In the context of change point detection for dynamic network models, we will describing the impact of hidden long range dependence on the evolution of such models (Joint work with Sayan Banerjee, Iain Carmichael, Jimmy Jin and Andrew Nobel). 3. In the context of attributed network models, we will describe what a theoretical analysis say about the behavior of centrality mechanisms such as page rank and degree centrality and the surprising efficacy of page rank driven sampling in the specific setting of sampling from rare minorities (Joint work with Nelson Antunes, Sayan Banerjee, and Vladas Pipiras).
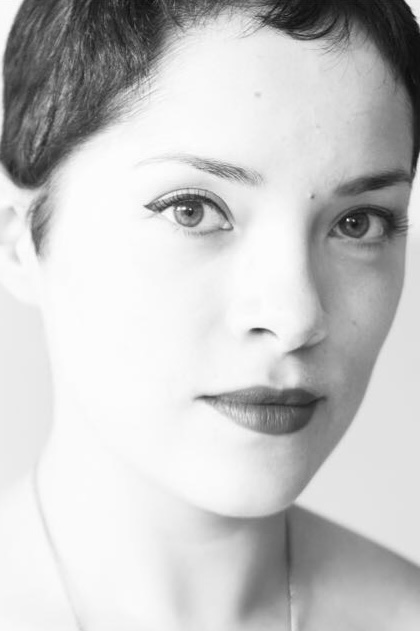
Social inequalities in ballet: implications for career success
by Yessica Herrera
07.03.2024 11am CET
Abstract. Global economic inequality remains a complex social challenge, impacting various facets of our society, including the arts. There is growing evidence suggesting inequalities in artists’ access to economic growth and leading positions in their careers. Hence, understanding the social dynamics of inequalities within the arts has become increasingly crucial. In particular, performing arts have long mirrored and perpetuated social inequalities, originating among white male aristocrats. Today, these disparities persist as systemic unequal access to institutional prestige and a disadvantaged professional environment for women. Recent decades have seen significant improvements in quantifying the behaviors and impact of scientists through the development of new methodologies, such as network science and the science of science, revealing patterns underlying successful careers. While performance in the arts has long been difficult to quantify objectively, research suggests that professional networks and prestige of affiliations play an important role in predicting career success, similar to observations in science. In this talk, we turn our attention to ballet—a mainstream performing art steeped in historical inequalities—as it allows us to investigate the interplay of individual performance, institutional prestige, and network effects quantitatively. We analyze data on competition outcomes from 6363 ballet students affiliated with 1603 schools in the United States, who participated in the Youth America Grand Prix (YAGP) between 2000 and 2021. Through network analysis, multiple logit models, and matching experiments, we provide evidence that schools’ strategic network position bridging between communities captures social prestige and predicts the placement of students into jobs in ballet companies. This work highlights the importance of institutional prestige on career success in ballet and showcases the potential of network science approaches to offer quantitative perspectives for career development beyond science. Moreover, network science research has proven useful in shedding light on the social components of gender inequalities in the field, emphasizing the need for more collaborative efforts to address systemic inequalities within the arts in future research.
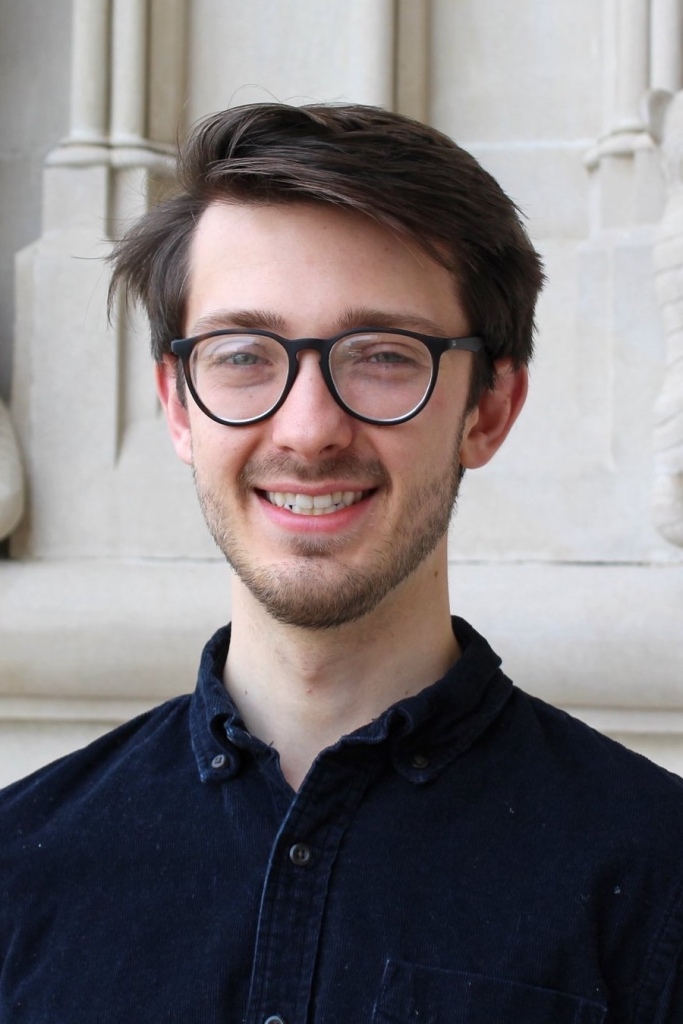
Human-Algorithm Decision-Making Under Imperfect Proxy Labels
by Luke Guerdan
29.02.2024 2pm CET
Abstract. Across domains such as medicine, employment, and social media, predictive models often target labels that imperfectly reflect the outcomes of interest to experts and policymakers. For example, clinical risk assessments deployed to inform physician decision-making often predict measures of healthcare utilization (e.g., costs, hospitalization) as a proxy for patient medical need. These proxies can be subject to outcome measurement error when they systematically differ from the target outcome they are intended to measure. In this talk, we discuss five sources of target variable bias that can impact the validity of proxy labels in human-algorithm decision-making tasks. We develop a causal framework to disentangle the relationship between each bias and clarify which are of concern in specific decision-making settings. We first leverage our framework to re-examine the designs of prior human subjects experiments that investigate human-algorithm decision-making and find that only a small fraction examine factors related to target variable bias. Next, we propose an algorithmic technique which, given knowledge of proxy measurement error properties, corrects for the combined effects of these challenges. We demonstrate the utility of our approach via experiments on real-world data from randomized controlled trials conducted in healthcare and employment domains. Our work underscores the importance of considering intersectional threats to model validity during the design and evaluation of human-algorithm decision-making workflows. We conclude by discussing the implications of imperfect proxy labels on efforts to adequately measure and mitigate network inequality.
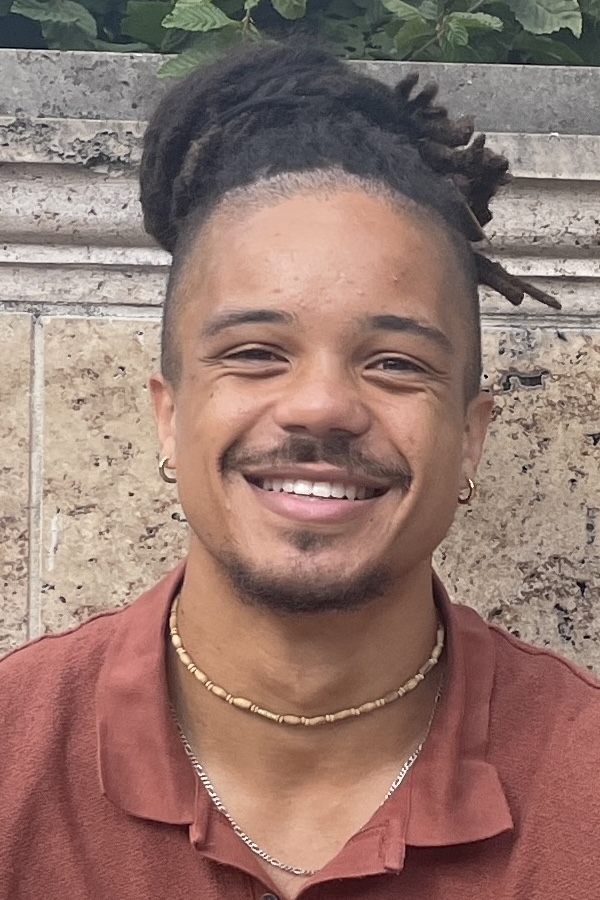
Cooperation and Inequality in Stochastic Models of Growth
by Jordan Kemp
15.02.2024 3pm CET
Absract. Group formation and collective action are fundamental to cooperative agents seeking to maximize resource growth. Researchers have extensively explored social interaction structures via game theory and homophilic linkages, such as kin selection and scalar stress, to understand emergent cooperation in complex systems. However, we still lack a general theory capable of predicting how agents benefit from heterogeneous preferences, joint information, or skill complementarities in statistical environments. In this talk, we derive general statistical dynamics for the origin of growth and cooperation based on the management of resources and pooled information. Specifically, we show how groups that optimally combine complementary agent knowledge in statistical environments maximize their growth rate. We show that these advantages are quantified by the information synergy embedded in the conditional probability of environmental states given agents’ signals, such that groups with a greater diversity of signals maximize their collective information. It follows that, when constraints are placed on group formation, agents must intelligently select with whom they cooperate to maximize the synergy available to their own signal. We then show how heterogeneity across groups drives resource inequality, which can be mitigated across similar groups through learning in shared environments. These results show how the general properties of information underlie optimal collective formation and drive the emergence of inequality in social systems.
Disclaimer: The list of speakers for this lecture series was compiled through an open nomination process that took place from December 11, 2023, to January 24, 2024. We received a total of 19 nominations, with a diverse pool of 9 men and 10 women. The final list of confirmed speakers includes 9 men and 8 women. We are committed to transparency and equal opportunities in our speaker selection process, and we encourage nominations from all qualified individuals regardless of gender, race, ethnicity, or any other protected characteristic or social capital.